Introduction to swapper
Milan Malfait
Ghent Universitymilan.malfait94@gmail.com
25 February 2025
Source:vignettes/swapper.Rmd
swapper.Rmd
Introduction
The swapper package implements a simple DE simulator based on feature swapping.The essence of the method involves randomly scrambling a subset of features in one subgroup of the data. This effectively induces a DE signal for those scrambled genes, while retaining the characteristics of the original data set without having to rely on any modeling assumptions.
Quick start
Mock up a data set
First we mock up a dataset.
sce <- scuttle::mockSCE(ncells = 100, ngenes = 50)
sce
#> class: SingleCellExperiment
#> dim: 50 100
#> metadata(0):
#> assays(1): counts
#> rownames(50): Gene_0001 Gene_0002 ... Gene_0049 Gene_0050
#> rowData names(0):
#> colnames(100): Cell_001 Cell_002 ... Cell_099 Cell_100
#> colData names(3): Mutation_Status Cell_Cycle Treatment
#> reducedDimNames(0):
#> mainExpName: NULL
#> altExpNames(1): Spikes
This mock data set contains a "Treatment"
variable,
which is just a random partitioning of the cells in 2 groups.
table(sce$Treatment)
#>
#> treat1 treat2
#> 53 47
Simulate DE by swapping features
Next we use swapper to simulate DE genes,
using the "Treatment"
variable as the grouping factor. We
induce DE in 10% of the original genes for cells belonging to the
"treat1"
group.
set.seed(123) # for reproducibility
cells_to_swap <- sce$Treatment == "treat1"
sim <- simulateDE(sce, which_cols = cells_to_swap, prop_DE = 0.1)
rowData(sim)
#> DataFrame with 50 rows and 2 columns
#> is_DE swapped_feature
#> <logical> <character>
#> Gene_0001 FALSE NA
#> Gene_0002 FALSE NA
#> Gene_0003 TRUE Gene_0031
#> Gene_0004 FALSE NA
#> Gene_0005 FALSE NA
#> ... ... ...
#> Gene_0046 FALSE NA
#> Gene_0047 FALSE NA
#> Gene_0048 FALSE NA
#> Gene_0049 FALSE NA
#> Gene_0050 FALSE NA
table(rowData(sim)$is_DE)
#>
#> FALSE TRUE
#> 46 4
Explore the simulated data
From the rowData
we can retrieve the true DE genes. The
rowData
also contains information regarding which gene was
swapped with which.
(trueDE_genes <- rownames(sim)[rowData(sim)$is_DE])
#> [1] "Gene_0003" "Gene_0015" "Gene_0031" "Gene_0042"
rowData(sim)[trueDE_genes, ]
#> DataFrame with 4 rows and 2 columns
#> is_DE swapped_feature
#> <logical> <character>
#> Gene_0003 TRUE Gene_0031
#> Gene_0015 TRUE Gene_0042
#> Gene_0031 TRUE Gene_0015
#> Gene_0042 TRUE Gene_0003
The "sim_group"
column in the colData
indicates for which cells the swapping occurred. In this example, this
should be equivalent to the "Treatment"
grouping.
colData(sim)
#> DataFrame with 100 rows and 4 columns
#> Mutation_Status Cell_Cycle Treatment sim_group
#> <character> <character> <character> <logical>
#> Cell_001 negative G0 treat1 TRUE
#> Cell_002 negative G0 treat1 TRUE
#> Cell_003 negative G2M treat1 TRUE
#> Cell_004 positive S treat1 TRUE
#> Cell_005 negative G0 treat2 FALSE
#> ... ... ... ... ...
#> Cell_096 negative G1 treat1 TRUE
#> Cell_097 negative G2M treat1 TRUE
#> Cell_098 positive G0 treat1 TRUE
#> Cell_099 negative G1 treat1 TRUE
#> Cell_100 positive G0 treat2 FALSE
table(sim$Treatment, sim$sim_group)
#>
#> FALSE TRUE
#> treat1 0 53
#> treat2 47 0
We can visualize these and compare them to their original counts to see how the swapping works.
if (requireNamespace("scater", quietly = TRUE)) {
library(scater)
## Log-normalize counts for visualization
sce <- logNormCounts(sce)
sim <- logNormCounts(sim)
p_orig <- plotExpression(
sce, features = trueDE_genes, x = "Treatment", colour_by = "Treatment"
) + ggtitle("Original counts")
p_sim <- scater::plotExpression(
sim, features = trueDE_genes, x = "Treatment", colour_by = "Treatment"
) + ggtitle("Simulated counts")
gridExtra::grid.arrange(p_orig, p_sim, ncol = 2)
}
#> Loading required package: scuttle
#> Loading required package: ggplot2
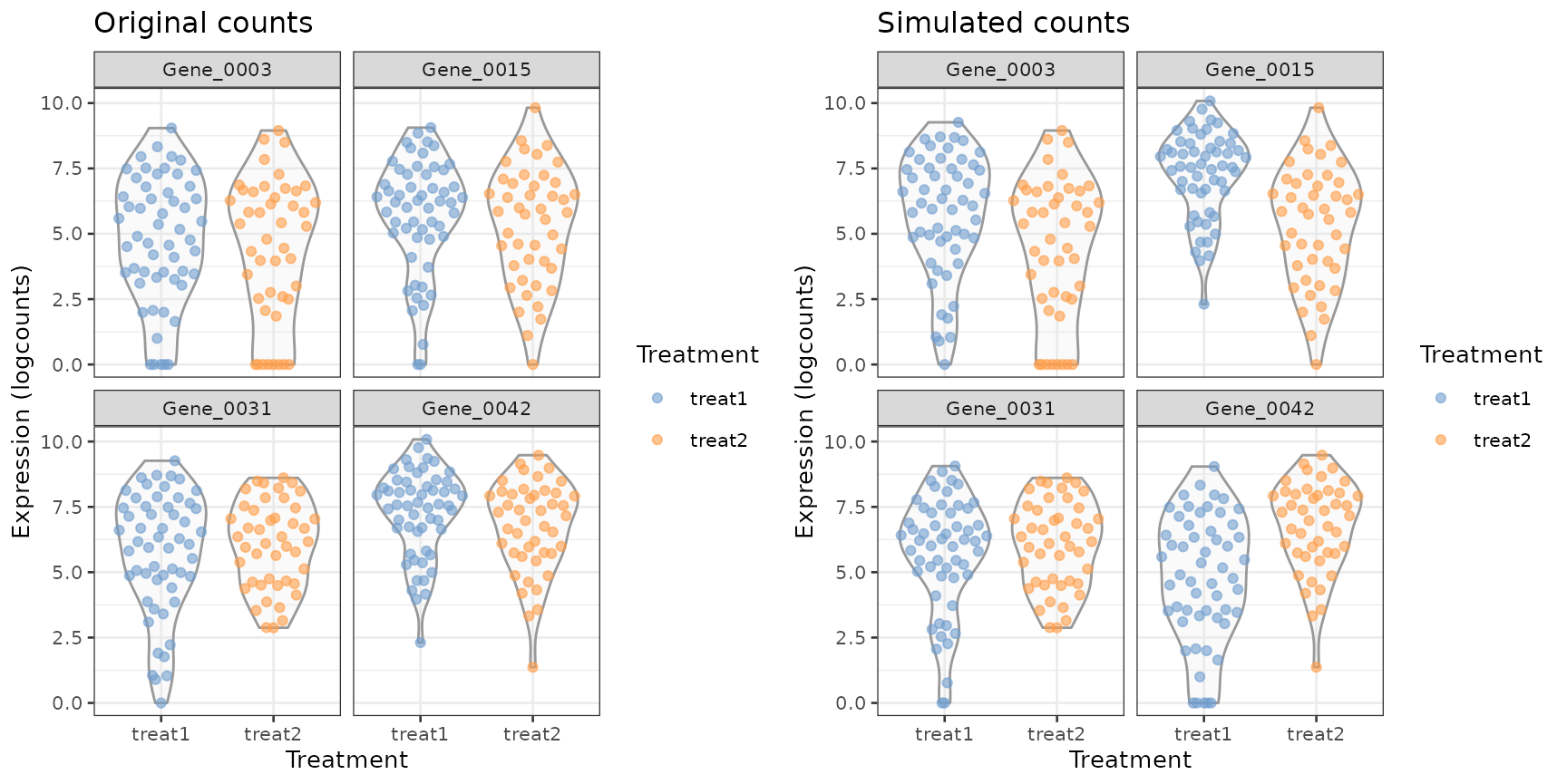
Comparing expression values for the simulated DE genes between the original data (left) and simulated data (right).
From this plot and the rowData
shown above, we can see
that the counts for e.g. Gene_0003
in the
treat1
group of the simulated data originated from the
treat1
counts for Gene_0014
in the original
data. Likewise, the simulated treat1
counts for
Gene_0014
are the original treat1
counts for
Gene_0042
. So we effectively induced DE by selectively
swapping out counts in one, but not the other, treatment group.
Session Info
Session info
#> ─ Session info ───────────────────────────────────────────────────────────────────────────────────────────────────────
#> setting value
#> version R version 4.4.2 (2024-10-31)
#> os Ubuntu 24.04.1 LTS
#> system x86_64, linux-gnu
#> ui X11
#> language en
#> collate C.UTF-8
#> ctype C.UTF-8
#> tz UTC
#> date 2025-02-25
#> pandoc 3.1.11 @ /opt/hostedtoolcache/pandoc/3.1.11/x64/ (via rmarkdown)
#> quarto NA
#>
#> ─ Packages ───────────────────────────────────────────────────────────────────────────────────────────────────────────
#> package * version date (UTC) lib source
#> abind 1.4-8 2024-09-12 [1] RSPM
#> beachmat 2.22.0 2024-10-29 [1] Bioconduc~
#> beeswarm 0.4.0 2021-06-01 [1] RSPM
#> Biobase * 2.66.0 2024-10-29 [1] Bioconduc~
#> BiocGenerics * 0.52.0 2024-10-29 [1] Bioconduc~
#> BiocManager 1.30.25 2024-08-28 [1] RSPM
#> BiocNeighbors 2.0.1 2024-11-28 [1] Bioconduc~
#> BiocParallel 1.40.0 2024-10-29 [1] Bioconduc~
#> BiocSingular 1.22.0 2024-10-29 [1] Bioconduc~
#> BiocStyle * 2.34.0 2024-10-29 [1] Bioconduc~
#> bookdown 0.42 2025-01-07 [1] RSPM
#> bslib 0.9.0 2025-01-30 [1] RSPM
#> cachem 1.1.0 2024-05-16 [1] RSPM
#> cli 3.6.4 2025-02-13 [1] RSPM
#> codetools 0.2-20 2024-03-31 [3] CRAN (R 4.4.2)
#> colorspace 2.1-1 2024-07-26 [1] RSPM
#> crayon 1.5.3 2024-06-20 [1] RSPM
#> DelayedArray 0.32.0 2024-10-29 [1] Bioconduc~
#> desc 1.4.3 2023-12-10 [1] RSPM
#> digest 0.6.37 2024-08-19 [1] RSPM
#> evaluate 1.0.3 2025-01-10 [1] RSPM
#> farver 2.1.2 2024-05-13 [1] RSPM
#> fastmap 1.2.0 2024-05-15 [1] RSPM
#> fs 1.6.5 2024-10-30 [1] RSPM
#> GenomeInfoDb * 1.42.3 2025-01-27 [1] Bioconduc~
#> GenomeInfoDbData 1.2.13 2025-02-25 [1] Bioconductor
#> GenomicRanges * 1.58.0 2024-10-29 [1] Bioconduc~
#> ggbeeswarm 0.7.2 2023-04-29 [1] RSPM
#> ggplot2 * 3.5.1 2024-04-23 [1] RSPM
#> ggrepel 0.9.6 2024-09-07 [1] RSPM
#> glue 1.8.0 2024-09-30 [1] RSPM
#> gridExtra 2.3 2017-09-09 [1] RSPM
#> gtable 0.3.6 2024-10-25 [1] RSPM
#> htmltools 0.5.8.1 2024-04-04 [1] RSPM
#> httr 1.4.7 2023-08-15 [1] RSPM
#> IRanges * 2.40.1 2024-12-05 [1] Bioconduc~
#> irlba 2.3.5.1 2022-10-03 [1] RSPM
#> jquerylib 0.1.4 2021-04-26 [1] RSPM
#> jsonlite 1.9.0 2025-02-19 [1] RSPM
#> knitr 1.49 2024-11-08 [1] RSPM
#> labeling 0.4.3 2023-08-29 [1] RSPM
#> lattice 0.22-6 2024-03-20 [3] CRAN (R 4.4.2)
#> lifecycle 1.0.4 2023-11-07 [1] RSPM
#> magrittr 2.0.3 2022-03-30 [1] RSPM
#> Matrix 1.7-1 2024-10-18 [3] CRAN (R 4.4.2)
#> MatrixGenerics * 1.18.1 2025-01-09 [1] Bioconduc~
#> matrixStats * 1.5.0 2025-01-07 [1] RSPM
#> munsell 0.5.1 2024-04-01 [1] RSPM
#> pillar 1.10.1 2025-01-07 [1] RSPM
#> pkgconfig 2.0.3 2019-09-22 [1] RSPM
#> pkgdown 2.1.1 2024-09-17 [1] any (@2.1.1)
#> R6 2.6.1 2025-02-15 [1] RSPM
#> ragg 1.3.3 2024-09-11 [1] RSPM
#> Rcpp 1.0.14 2025-01-12 [1] RSPM
#> rlang 1.1.5 2025-01-17 [1] RSPM
#> rmarkdown 2.29 2024-11-04 [1] RSPM
#> rsvd 1.0.5 2021-04-16 [1] RSPM
#> S4Arrays 1.6.0 2024-10-29 [1] Bioconduc~
#> S4Vectors * 0.44.0 2024-10-29 [1] Bioconduc~
#> sass 0.4.9 2024-03-15 [1] RSPM
#> ScaledMatrix 1.14.0 2024-10-29 [1] Bioconduc~
#> scales 1.3.0 2023-11-28 [1] RSPM
#> scater * 1.34.0 2024-10-29 [1] Bioconduc~
#> scuttle * 1.16.0 2024-10-29 [1] Bioconduc~
#> sessioninfo 1.2.3 2025-02-05 [1] RSPM
#> SingleCellExperiment * 1.28.1 2024-11-10 [1] Bioconduc~
#> SparseArray 1.6.2 2025-02-20 [1] Bioconduc~
#> SummarizedExperiment * 1.36.0 2024-10-29 [1] Bioconduc~
#> swapper * 0.99.2 2025-02-25 [1] local
#> systemfonts 1.2.1 2025-01-20 [1] RSPM
#> textshaping 1.0.0 2025-01-20 [1] RSPM
#> tibble 3.2.1 2023-03-20 [1] RSPM
#> UCSC.utils 1.2.0 2024-10-29 [1] Bioconduc~
#> vctrs 0.6.5 2023-12-01 [1] RSPM
#> vipor 0.4.7 2023-12-18 [1] RSPM
#> viridis 0.6.5 2024-01-29 [1] RSPM
#> viridisLite 0.4.2 2023-05-02 [1] RSPM
#> withr 3.0.2 2024-10-28 [1] RSPM
#> xfun 0.51 2025-02-19 [1] RSPM
#> XVector 0.46.0 2024-10-29 [1] Bioconduc~
#> yaml 2.3.10 2024-07-26 [1] RSPM
#> zlibbioc 1.52.0 2024-10-29 [1] Bioconduc~
#>
#> [1] /home/runner/work/_temp/Library
#> [2] /opt/R/4.4.2/lib/R/site-library
#> [3] /opt/R/4.4.2/lib/R/library
#> * ── Packages attached to the search path.
#>
#> ──────────────────────────────────────────────────────────────────────────────────────────────────────────────────────